Laboratorul 4
(Deadline: 30.05.2025 23:59:59)
Daca nu sunteti logati exercitiile nu se mai afiseaza.
NLTK
NLTK (Natural Language Toolkit) este o platforma specializata pe procesarea limbajului natural. Pachetul NLTK implementeaza cei mai cunoscuti algoritmi din acest domeniu, si, in plus vine si cu o semnificativa parte de date, continand corpusurile cele mai utilizate.
Instalarea NLTK
Pentru instalarea NLTK vom folosi pip
.
Scrieti in linia de comanda
pip install nltk
Dupa instalare putem importa modulul in consola:
import nltk
Descarcati resursele pentru nltk (ideal ar fi pachetele pentru limba engleza + datele din book - sunt niste resurse folosite in manualul principal despre NLTK, dar putem sa le folosim si noi in aplicatii).
nltk.download()
In urma apelului functiei download ar trebui sa vi se afiseze un ecran de forma: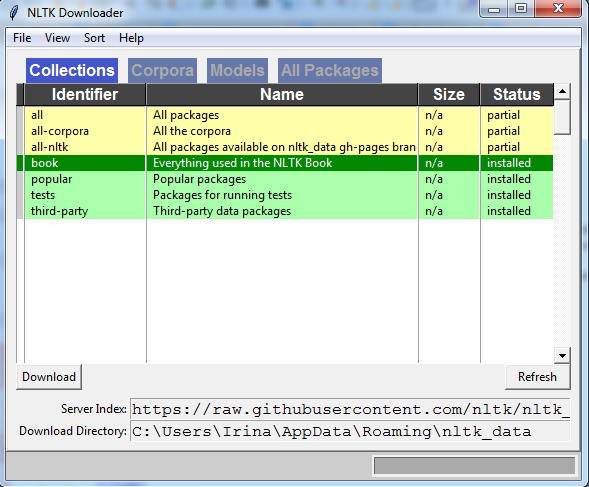
Observati ca sunt mai multe taburi:
- Collections - este cu colectiile mari de pachete (un fel de afisare compacta)
- Corpora - este afisarea detaliata a pachetelor cu corpusuri - din care alegeti voi ce aveti nevoie
- Models (date)
- All packages - pachetele afisate detaliat.
Texte predefinite
In exercitiile de laborator vom folosi textele din modulul book (si alte cateva tool-uri de acolo). Pentru a le importa, scriem:
>>> from nltk.book import *
Ar trebui sa vedeti un output asemanator cu cel de mai sus.
*** Introductory Examples for the NLTK Book ***
Loading text1, ..., text9 and sent1, ..., sent9
Type the name of the text or sentence to view it.
Type: 'texts()' or 'sents()' to list the materials.
text1: Moby Dick by Herman Melville 1851
text2: Sense and Sensibility by Jane Austen 1811
text3: The Book of Genesis
text4: Inaugural Address Corpus
text5: Chat Corpus
text6: Monty Python and the Holy Grail
text7: Wall Street Journal
text8: Personals Corpus
text9: The Man Who Was Thursday by G . K . Chesterton 1908
>>>
Analiza morfologica
Pentru a realiza analiza morfologica, vom folosi POS taggerul (Part Of ) de la Stanford: https://nlp.stanford.edu/software/. Indicat este sa descarcati versiunea full. Dezarhivati taggerul si observati un jar cu numele de forma stanford-postagger.jar.
Importati in consola taggerul:
from nltk.tag.stanford import StanfordPOSTagger
Vrem sa ne creem o instanta a taggerului:
tagger=StanfordPOSTagger(cale_model, cale_jar_tagger)
Pentru a aplica tagurile POS folosim metoda tag()
tagger.tag(["I","saw","a","cat"])
In cazul in care primiti o eroare la incercarea folosirii taggerului, urmati indicatiile de aici: https://stackoverflow.com/questions/34692987/cant-make-stanford-pos-tagger-working-in-nltk
Incercati sa parsati o propozitie si urmariti semnificatia tagurilor
In cazul in care primim un text ca sir si nu ca lista de cuvinte, putem sa il tokenizam:
>>> nltk.word_tokenize("It was late. I saw a cat chasing a mouse.")
Stopwords:
['It', 'was', 'late', '.', 'I', 'saw', 'a', 'cat', 'chasing', 'a', 'mouse', '.']
>>>
>>> from nltk.corpus import stopwords
>>> stopwords.words('english')
['i', 'me', 'my', 'myself', 'we', 'our', 'ours', 'ourselves', 'you', "you're", "you've", "you'll", "you'd", 'your', 'yours', 'yourself', 'yourselves', 'he', 'him', 'his', 'himself', 'she', "she's", 'her', 'hers', 'herself', 'it', "it's", 'its', 'itself', 'they', 'them', 'their', 'theirs', 'themselves', 'what', 'which', 'who', 'whom', 'this', 'that', "that'll", 'these', 'those', 'am', 'is', 'are', 'was', 'were', 'be', 'been', 'being', 'have', 'has', 'had', 'having', 'do', 'does', 'did', 'doing', 'a', 'an', 'the', 'and', 'but', 'if', 'or', 'because', 'as', 'until', 'while', 'of', 'at', 'by', 'for', 'with', 'about', 'against', 'between', 'into', 'through', 'during', 'before', 'after', 'above', 'below', 'to', 'from', 'up', 'down', 'in', 'out', 'on', 'off', 'over', 'under', 'again', 'further', 'then', 'once', 'here', 'there', 'when', 'where', 'why', 'how', 'all', 'any', 'both', 'each', 'few', 'more', 'most', 'other', 'some', 'such', 'no', 'nor', 'not', 'only', 'own', 'same', 'so', 'than', 'too', 'very', 's', 't', 'can', 'will', 'just', 'don', "don't", 'should', "should've", 'now', 'd', 'll', 'm', 'o', 're', 've', 'y', 'ain', 'aren', "aren't", 'couldn', "couldn't", 'didn', "didn't", 'doesn', "doesn't", 'hadn', "hadn't", 'hasn', "hasn't", 'haven', "haven't", 'isn', "isn't", 'ma', 'mightn', "mightn't", 'mustn', "mustn't", 'needn', "needn't", 'shan', "shan't", 'shouldn', "shouldn't", 'wasn', "wasn't", 'weren', "weren't", 'won', "won't", 'wouldn', "wouldn't"]
>>>